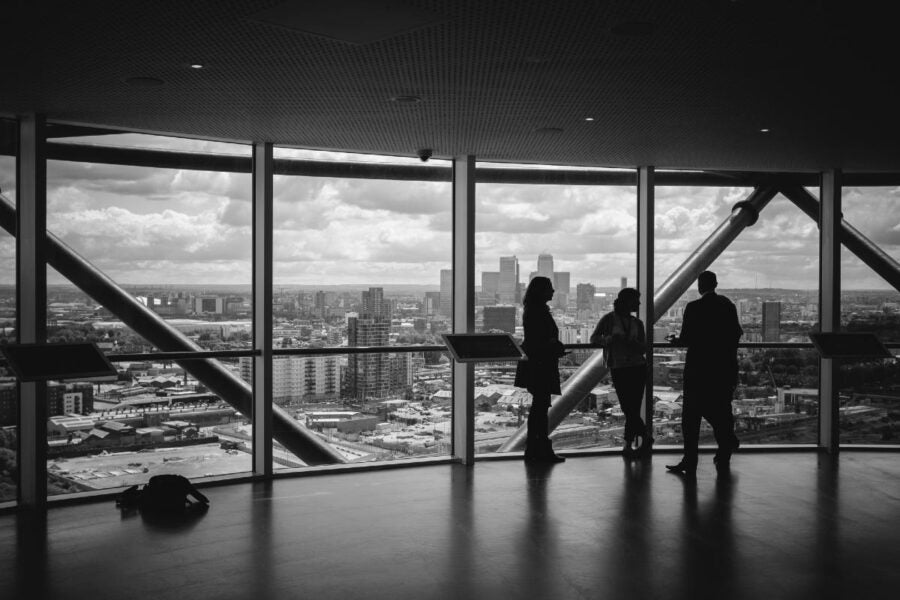
The meteoric rise of GenAI has ushered in a new age of disruptive innovation. Just eighteen months after ChatGPT blazed a trail for the technology, thousands of businesses are now using it to unlock fresh insights, automate mundane tasks and create new content. Even firms that haven’t yet embraced AI can’t deny its impact; we are clearly on the cusp of a technological shift that will rival – and potentially exceed – the introduction of the internet or mobile devices.
“You can’t ignore it,” says Steven Huels, general manager of the AI business unit at Red Hat, a leading provider of enterprise open-source solutions. “It’s not going to go away. It’s going to have far-reaching impacts on your business, your competitive nature, [etc.]”
Indeed, Gartner estimates that 85% of enterprises will have used GenAI application programming interfaces (APIs) or deployed GenAI-enabled applications by 2026. Nevertheless, many organisations are struggling to get projects into production quickly – and crucially cost-effectively. Often that’s because they lack the talent, partners or tools to successfully enhance their applications with AI.
The lack of alignment between rapidly evolving tools can lower productivity and complicate collaboration between data scientists, software developers and IT operations, for instance. Complex administrative processes may further undermine efforts to scale AI deployments. While popular cloud platforms seem to offer the scalability and attractive toolsets enterprises need, they often come with a significant degree of user lock-in, which can limit architectural and deployment options.
To achieve the kind of scale that will deliver real value, companies also need to ensure repeatable and consistent handoffs between model developers, application developers and operations, along with effective AI lifecycle management.
“If you’re building an enterprise application, that code is managed, it has a lifecycle – there’s a roadmap for how it grows and how it impacts your business,” says Huels. “[It’s] the same philosophy for enterprise AI.”
Finding the right approach
Given how quickly the AI landscape is evolving, it’s perhaps not surprising that many enterprises are still figuring out how to deploy and scale the technology. Fine-tuning a foundation model with company data once seemed like the best approach, for example. But considerable funds, time and data expertise are needed to achieve this – something many organisations lack.
Although fine-tuning is often very effective when it comes to meeting a specific AI use case, retrieval augmented generation (RAG), which enhances the accuracy and reliability of generative AI models with facts drawn from an external knowledge base, allows enterprises to incorporate data into a pre-trained model in a faster and more cost-effective way.
“Every customer has a knowledge base,” says Huels. “Whether it’s a product knowledge base, a customer knowledge base, a support knowledge base – they have this readily available. With RAG they don’t have to burn their data into the model, so it gives them the ability to try different models as they are emerging and swap them out.”
you need a core platform that allows you to make bets without compromising your entire data centre and AI strategy
Regardless of which approach they adopt, the fast-moving nature of AI means that enterprises will still need to make some speculative bets on emerging tools, partners and technologies in this space.
“Some [bets] will pay off, some won’t,” says Huels. “But underneath that you need a core platform that allows you to make bets without compromising your entire data centre and AI strategy.”
Red Hat’s OpenShift AI is designed to be that core platform. It provides organisations with an environment and set of tools to create AI applications for unique use cases and deploy them at scale across hybrid cloud environments. IT, data science and development teams can quickly access core AI libraries and frameworks and collaborate with ease, for example, helping to simplify projects and accelerate timelines.
OpenShift AI also provides IT operations with a security-focused platform that is simple to monitor and manage. The modular, open-source nature of the platform stands in contrast to the more prescriptive AI suites available from the big cloud providers too. And it can easily be extended with partner tools that will further enhance AI development and deployment.
“You get consistency in deployment, but you’re giving your end users a lot of choice in which tools they can use,” Huels explains. “So, if your developers prefer a no-code/ low-code model development environment, [for example], you can plug that into our platform.”
Open-source innovation
This should set enterprises up for a future where GenAI models are integrated into ever more applications and environments.
“You’re going to see increasing advancements on making these generative AI models smaller, more accessible, [and] able to run in environments that don’t require extensive capital outlays,” says Huels. Many of the innovations that drive this shift will be open source.
“There’s no denying the role open source plays not just in the model side, but the framework side, the development side,” says Huels. “It is going to be the key driver of AI innovation going forward.”
The open-source community is also likely to play a key role in the development of AI standards too. “The reason Enterprise Linux did so well was because a lot of it was built in the open,” says Huels.
“You had multiple eyes from varying backgrounds looking at the same sets of code, making sure things were operating the way they were supposed to – [that] they were optimised, they were secure. You’re starting to see that more with open model development.”
Regulations around AI are also likely to increase in future, though hopefully against a backdrop of better public understanding of the power and limitations of the technology.
Ultimately, says Huels, “We want that innovation to still occur, but we want it to occur in a way that [means] we have confidence that it [AI] is still acting in our best interest and not against us.”
No matter what the future holds though, platforms like OpenShift AI will clearly play a vital role in helping organisations to deploy and scale the technology at pace. In fact, says Huels, “Enterprises who refuse to adopt AI are going to find themselves in a spot where it’s really hard for them to compete against companies who are taking advantage of it.”
For more information please visit redhat.com
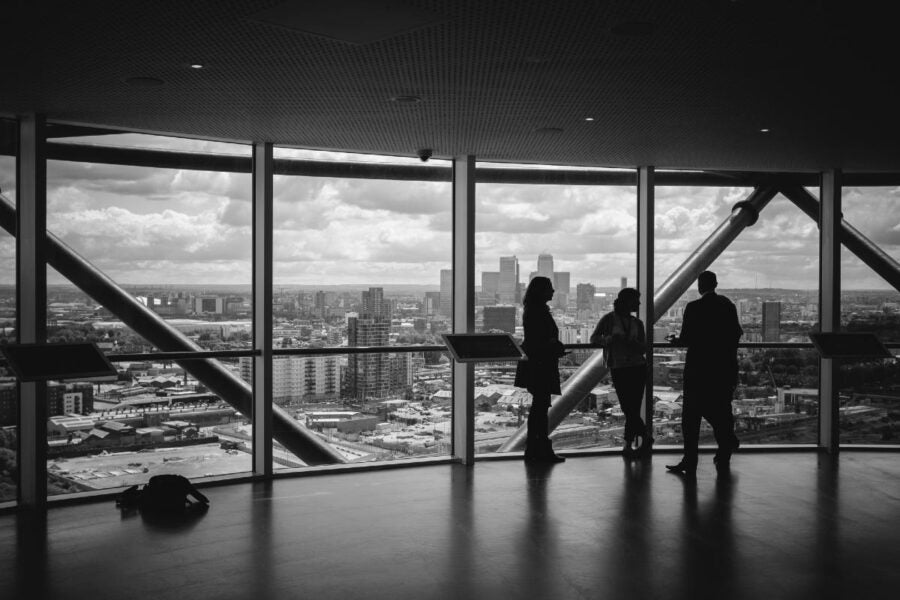
The meteoric rise of GenAI has ushered in a new age of disruptive innovation. Just eighteen months after ChatGPT blazed a trail for the technology, thousands of businesses are now using it to unlock fresh insights, automate mundane tasks and create new content. Even firms that haven’t yet embraced AI can’t deny its impact; we are clearly on the cusp of a technological shift that will rival – and potentially exceed – the introduction of the internet or mobile devices.
“You can’t ignore it,” says Steven Huels, general manager of the AI business unit at Red Hat, a leading provider of enterprise open-source solutions. “It’s not going to go away. It’s going to have far-reaching impacts on your business, your competitive nature, [etc.]”
Indeed, Gartner estimates that 85% of enterprises will have used GenAI application programming interfaces (APIs) or deployed GenAI-enabled applications by 2026. Nevertheless, many organisations are struggling to get projects into production quickly – and crucially cost-effectively. Often that’s because they lack the talent, partners or tools to successfully enhance their applications with AI.