What if, when the Fukushima nuclear plant was struck by a tsunami in 2011, the wind had blown onshore instead of out to sea? Or if a solar storm had hit during the London Olympics in 2012 — an event said to have a likelihood of 4 per cent?
These might sound like abstract thought experiments, but they are cited in a recent Lloyd’s of London report on so-called “counterfactual risk analysis”, a technique that involves imagining in detail how the past might have turned out differently to inform better risk modelling for businesses, insurers and risk managers.
Such work is part of a broader movement in risk analysis towards using big data, artificial intelligence and fresh approaches that are widely expected to yield vast improvements in forecasting, ultimately improving safety and reducing premiums.
Some insurers are already working on translating big data — enormous data sets that can be crunched to reveal new insights and trends — into predictive analytic tools that can, for example, identify the locations most likely to experience a loss, and identify equipment most likely to be to blame for a problem.
This means they can not only determine the severity of risk, but also where loss is most likely to occur — with accuracy never before possible.
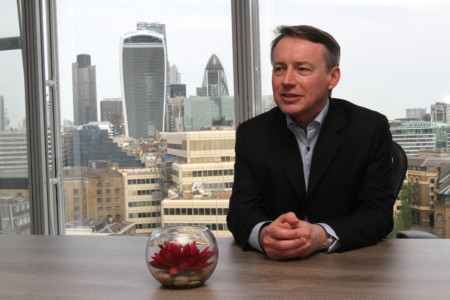
Colin Farquhar of Protiviti: legacy systems and data privacy are holding risk managers back
Colin Farquhar, a director in risk and compliance practice at Protiviti, a risk consultancy, says: “As more diverse data sets become available and more sophisticated computational algorithms analyse the data combinations, the ability to assess the likelihood of different outcomes will improve.”
The rise of connected devices and sensors, via the so-called internet of things, will also mean insurers will become much more attuned to changes that clients make at, say, manufacturing facilities, and whether those changes alter the risk profile, he adds.
Mr Farquhar offers an example of the benefits of improved risk modelling in the healthcare industry: anonymous data will facilitate the development of algorithms to “better predict longevity, medication outcomes, survival rates, time in hospital, readmission rates”, he says.
If more diverse data sets, and more sophisticated algorithms to analyse this data, are going to radically improve the ability to assess risk, is the “black swan” going to become an endangered species?
The term, coined by Nassim Nicholas Taleb, a finance professor, comes from the observation that black swans were thought to be extremely rare - until they were discovered living in vast numbers in Australia. It is now used to describe an extraordinary event that existing knowledge finds difficult to predict. Mr Farquhar suggests there is at least the potential for a reassessment of what qualifies as a black swan.
Improved predictions should lead to a lower frequency of extreme events and/or less severity when they occur
“Improved predictions should lead to a lower frequency of extreme events and/or less severity when they occur,” says Mr Farquhar. “They should also lead to better preparedness to deal with extreme events.”
What about a risk that, on the surface at least, arrives entirely out of the blue, such as the infamous series of industrial explosions in Tianjin, China, in 2015 which killed 173 people?
Reducing the risk of such a tragedy will partly depend on the willingness of business to invest in risk analysis. “In engineering terms, it is possible, given information about each component, to estimate the mean time to failure of an engine, as an example,” says Mr Farquhar. “If underlying component reliability was not well measured in general then the probability of extreme events across industries rises.”
However, he adds: “Extreme events, including unforeseen ones with substantial outcomes such as bankruptcy, cannot be totally prevented.
[xyz-ihs snippet=“2-FUTURE-OF-RISK-ANALYTICS-Black-swan-08”]
“There are always low probability but high impact events which may occur. Certainty is not possible and, if it were, there would be little opportunity to generate above risk-free returns,” Mr Farquhar points out.
There’s also caution over the extent to which businesses and risk managers are prepared to adopt the new approaches.
Paul Henninger, who runs the data and analytics practice at business advisory firm FTI Consulting, says: “Much of analytics that are used today are based on the idea of a ‘normal distribution’ where most things that happen are similar and very few unusual events happen out at the margins.”
“Understanding more about unusual events requires a different kind of math. Rare events are almost by definition marginal and you need to use an analytics approach that gives you information on the margins instead of on the most common events.”
Mr Farquhar says there is a wide variety of preparedness to embrace these new ways of analysing risk: “Some are forging ahead with leveraging this data while others have hardly started. Overall, developments are behind where they could be but much of this is driven by legacy system issues and sensitivities around data privacy.”
Those that don’t engage with the new approach to risk analysis risk being left behind, he warns: “Much of the technology and analytical techniques are quite different to that which have been used previously, so implementing such will involve considerable effort and organisational redesign to lead to effective outcomes.
“Firms which do not adapt are rapidly finding themselves disenfranchised by more disruptive entrants to their market but then have little time to react.”