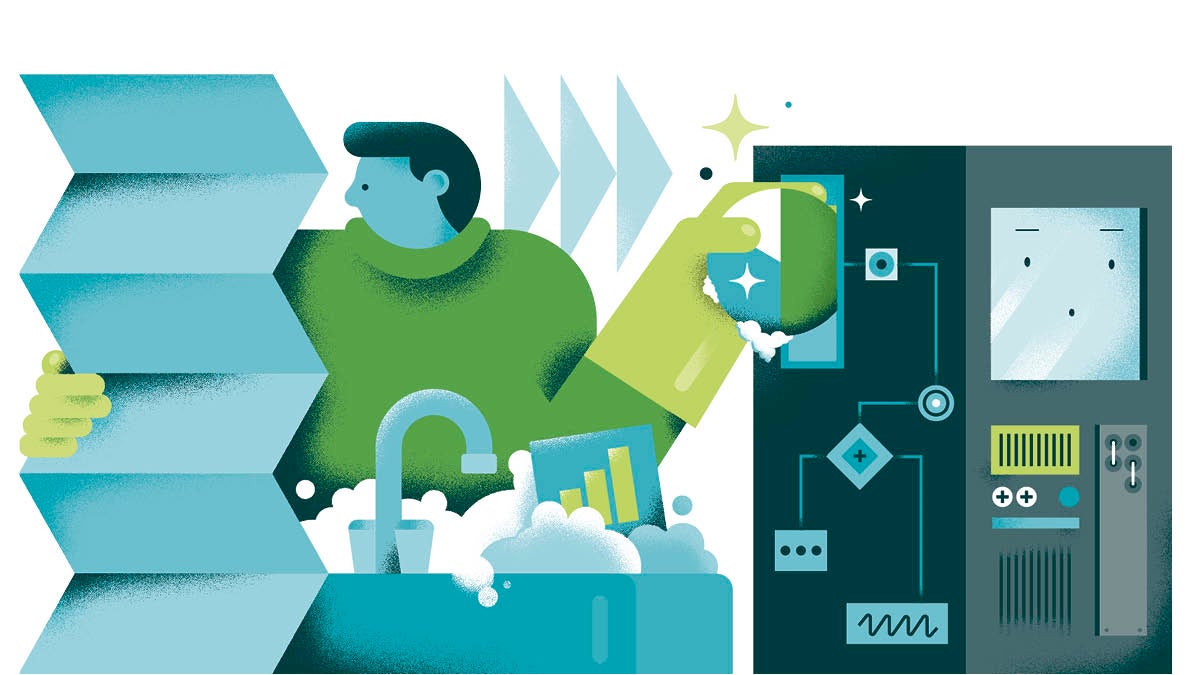
Are you prepared for AI implementation? Do you know what your accompanying data strategy should be? If not, it is likely you aren’t alone. According to research by Secondmind, 82 per cent of supply chain managers are frustrated by AI systems and tools during the coronavirus pandemic.
In its survey of 500-plus supply chain planners and managers across Europe and the United States, 37 per cent cited a lack of reliable data to feed into AI systems as a concern, at a time when accuracy and speed of decision-making were of the essence.
They don’t doubt AI’s capabilities; 90 per cent agreed AI will help them make better choices by 2025, but a third raised another critical issue in their leadership’s lack of understanding of what is currently needed to make faster, data-driven decisions.
So how do chief executives and the C-suite approach solving this? Listening to experts, most agree on the main problems. These include incomplete, dirty or duplicated data, siloed data, inherent bias in data programmed for AI models and a lack of focus or knowledge at board-level on what they hope AI can, and will, achieve.
Leila Seith Hassan, head of data at the UK arm of global marketing agency Digitas, believes it pays not to treat AI as a buzzword. She says: “This leads to a bit of naivety or even ignorance. Many don’t really understand what AI is or does and often apply a futuristic and/or simplified view.
Siloed data stores severely restrict AI’s ability to influence the digital ecosystem around it, rendering it little more than an expensive brain in a box
“Too often, expectations of AI are mandated without consideration of what’s feasible given an organisation’s data maturity. AI requires an organisation to have infrastructure, process and people in place before embarking on any serious project. If you don’t, you need to be prepared for the time and cost that comes with getting the organisation fit for purpose.
“Ultimately, AI is making decisions instead of humans. If you’re building your AI on bad data, it’s going to make bad decisions.”
Business decisions and processes must adapt
True AI implementation with the right data strategy requires investment, time, and the best and most experienced people. Get it right and the positives are clear, with increased profitability, productivity and reduced fraud among them.
Get it wrong, though, and things can be very different, especially if the data used fails to represent society or enforces existing biases. Trust and consent is also crucial to the process.
Dr Alan Bourne, chartered occupational psychologist and founder of Sova Assessment, explains: “When embedding AI into any business system, it is essential that a real person is placed front and centre of the process, so humanity, laws, regulations and ethics are considered with as much importance as technological capabilities. The opportunities to do this are vast, whether it be using an internal human resource, an advisory board or using AI to audit other forms of AI being applied to the business.”
Data-hungry algorithms must also be continually tested says Alix Melchy, vice president of AI at Jumio. “Another process that business must implement in their AI practices is a pilot testing phase, to ensure the algorithm is working as expected and to better understand why an algorithm is making a certain decision. By running a test in the early stages, and before the algorithm is put into the real-world scenario, feasibility, duration, cost and adverse events are all assessed,” he says.
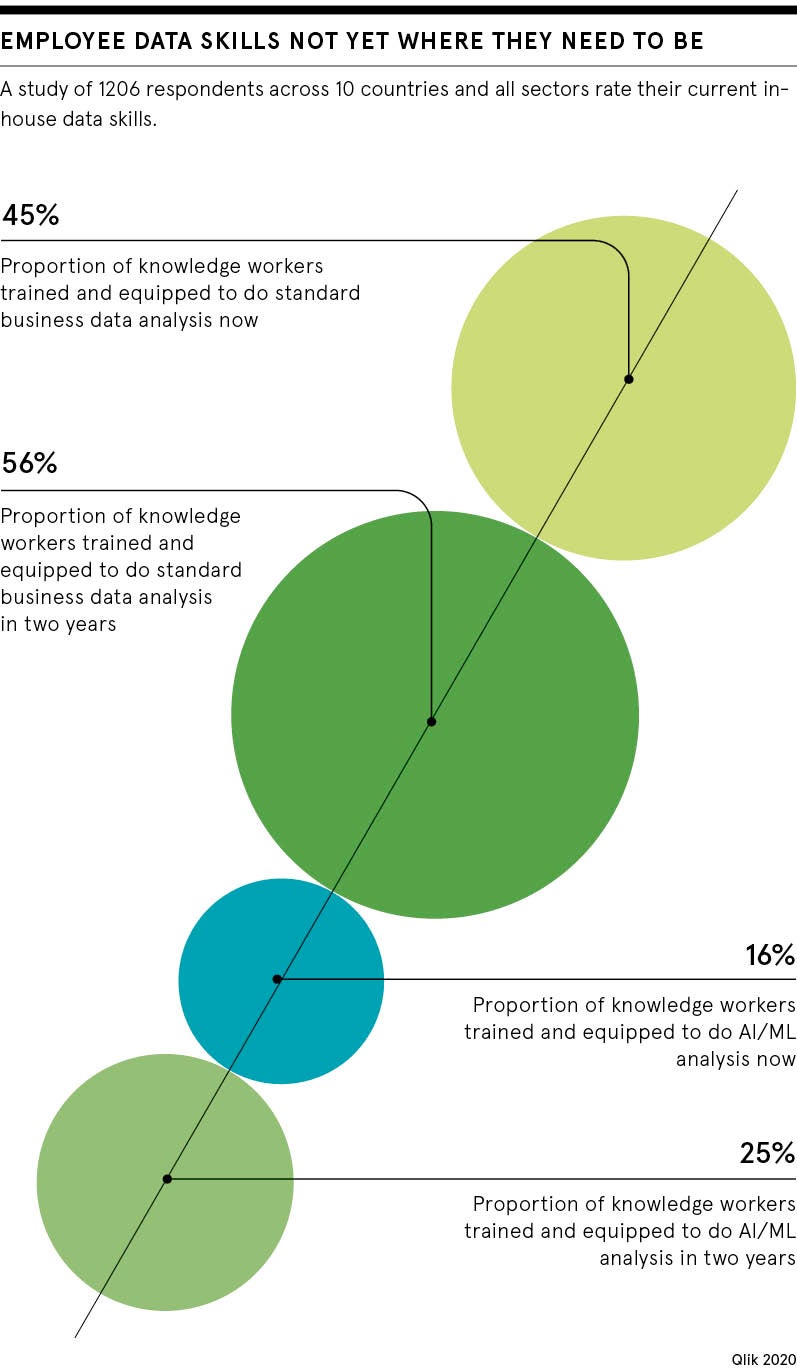
Clean, high-quality data critical to AI projects
Where the data comes from and how clean it is will be paramount in AI implementation. Historic data silos are often still needed for reasons of privacy and security, but this can cause problems, while the lack of a connected cloud solution serving all parts of the business can be a huge barrier too.
Paul Crerand, field chief technology officer for Europe, Middle East and Africa at MuleSoft, recommends an application programming interface strategy to easily connect any application, data source or device together over an app network, where data can flow freely.
“Siloed data stores and a lack of connectivity between enterprise applications severely restrict AI’s current ability to influence the digital ecosystem around it, rendering it little more than a rather expensive brain in a box,” he says.
AI requires an organisation to have infrastructure, process and people in place before embarking on any serious project
“Businesses must build a central nervous system that enables AI to plug in and out of any data source or capability that can provide or consume the intelligence it creates. Point-to-point integrations of the past will lead to atrophy in the AI-driven world, where things can change in an instant and even the near-future is uncertain. Organisations must decouple very complex systems and turn their data stores and digital capabilities into flexible, discoverable building blocks.”
Adrian Tam, director of data science at New York-based Synechron, offers a similar solution. “We have a term called ‘data lake’. It means to keep the data in its natural format in an accessible form. I think it doesn’t matter if the data is spread across servers and across geographic locations as long as we have a single, unified way to access it. So, if there are data silos, you just need to build an interface to use it,” he says.
“Of course, this is easier said than done because there are issues like back-up, version control, system resilience and availability. This is another engineering problem, but should not be part of the AI. It is a bad engineering practice to blend two problems into one unnecessarily.”
Dr Neil Yager, co-founder and chief scientist of Phrasee, addresses the cleansing of data. “It is not widely appreciated how much effort goes into data cleaning and preparation,” he says. “A model built using machine-learning is only as good as the data it was trained on. Poor quality data leads to poor quality models. Unfortunately, pristine data sets are rare in the wild; most datasets are riddled with problems.
“The sets are often distributed across multiple incompatible sources and missing or incorrect entries are common. A recent survey of data scientists concluded they spend around 45 per cent of their time on data preparation.”
AI implementation not just the CDO’s job
Combating all these challenges means having the right skills widely dispersed across an organisation to achieve AI implementation. A partnership approach between a traditional data scientist alongside a data engineer could be the answer, according to Dr Greg Benson, chief scientist at SnapLogic and professor of computer science at the University of San Francisco.
He says the former can “determine how to apply models and derive training examples from existing data sources” and the latter “understands how to navigate existing IT data systems, understands regulatory compliance considerations and ultimately knows how to build data pipelines”.
Elsewhere, research from Qlik with IDC showed just 16 per cent of knowledge workers globally are equipped to do AI and machine-learning analysis. This figure is predicted to rise to 25 per cent over the next two years, with the proportion of those with data literacy skills increasing from 45 to 63 per cent. Two-thirds in another Qlik study believed data literacy training would make them more productive.
Adam Mayer, senior manager at Qlik, says: “Many business leaders are recognising that having these capabilities siloed in business intelligence teams will prevent them from generating the greatest value from their data.”
Despite all the complication though, could the answer to AI implementation and data strategy be easier than we think? Jamie Hutton, chief technology officer of Quantexa, says: “There is usually a simple test: if there is not enough data for a human to make an accurate decision, then neither will the machine be able to do so.”
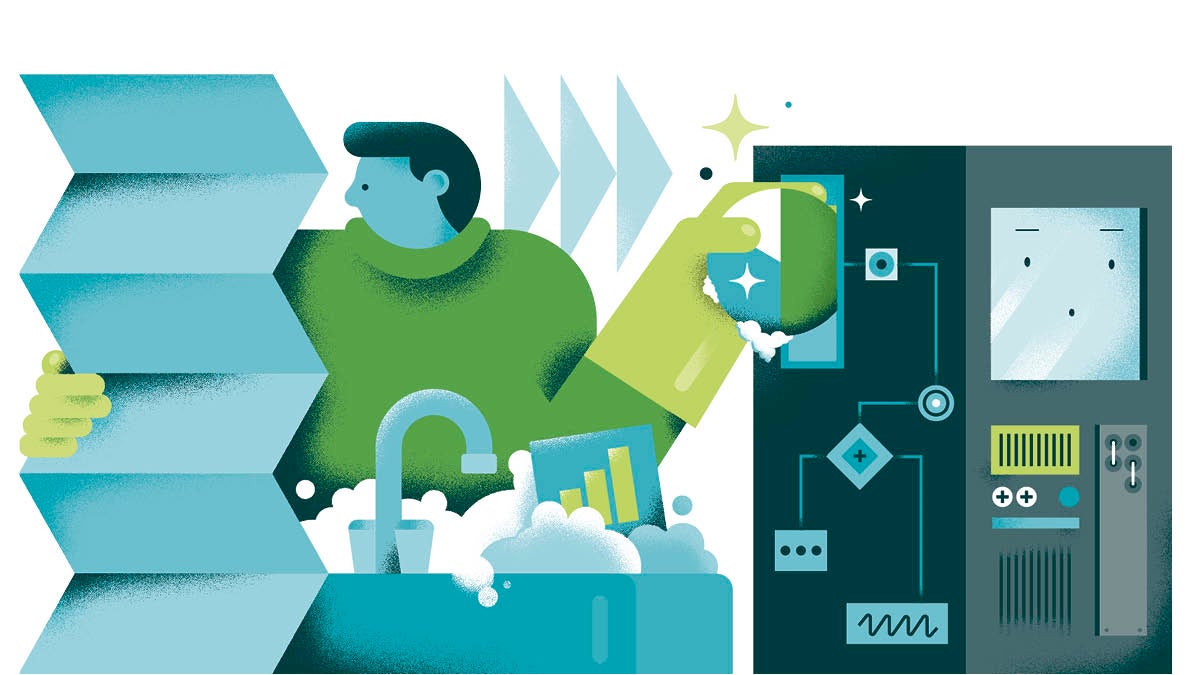
Are you prepared for AI implementation? Do you know what your accompanying data strategy should be? If not, it is likely you aren't alone. According to research by Secondmind, 82 per cent of supply chain managers are frustrated by AI systems and tools during the coronavirus pandemic.
In its survey of 500-plus supply chain planners and managers across Europe and the United States, 37 per cent cited a lack of reliable data to feed into AI systems as a concern, at a time when accuracy and speed of decision-making were of the essence.
They don't doubt AI's capabilities; 90 per cent agreed AI will help them make better choices by 2025, but a third raised another critical issue in their leadership’s lack of understanding of what is currently needed to make faster, data-driven decisions.