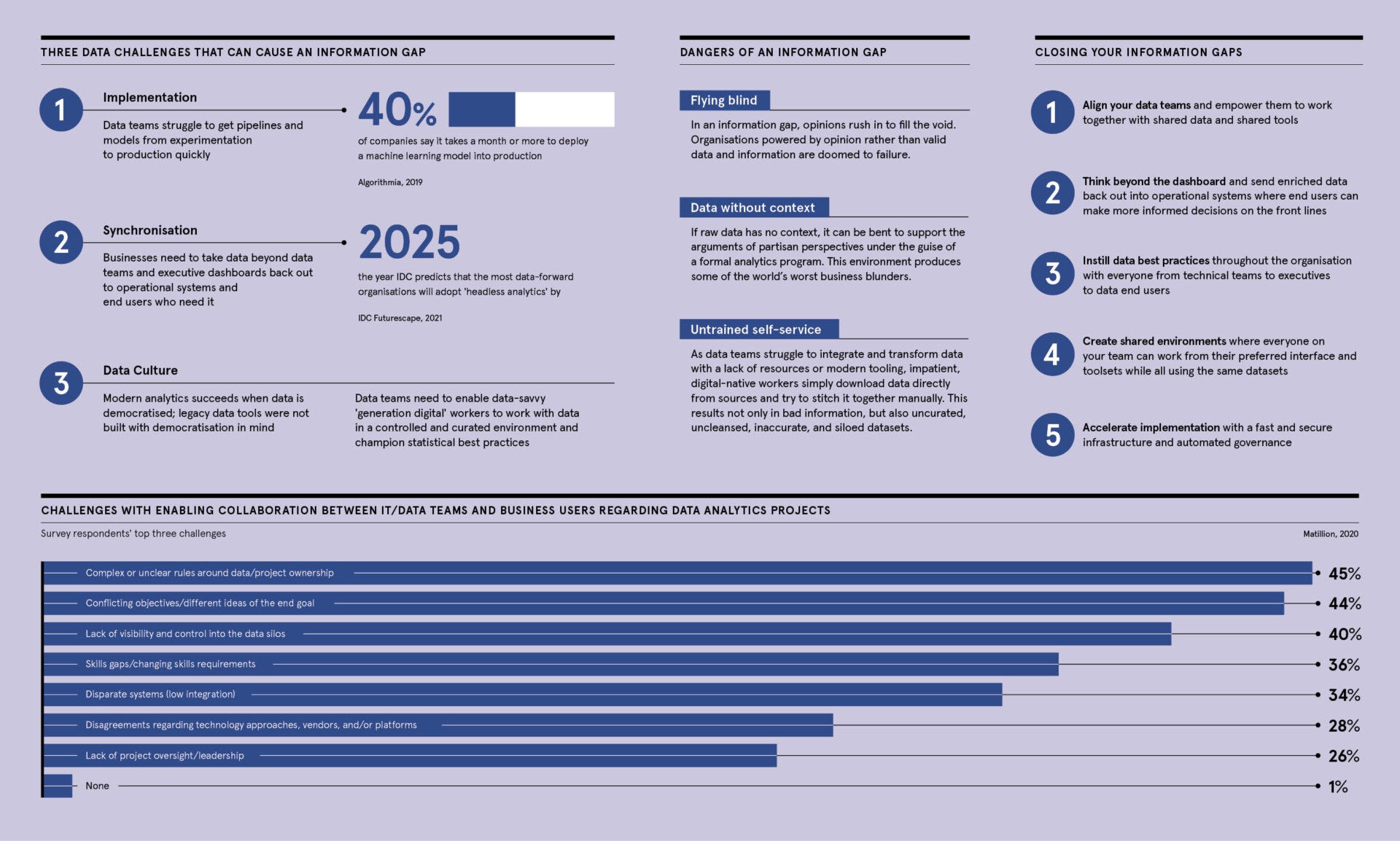
The world’s dependence on data has never been greater. Enterprises rely on analytics to take what are often huge volumes of raw data circulating through the business and transform it into rich, actionable information. However, with many organisations, this isn’t the case.
“Imagine you want to make a tool or a car or a ship or a bridge out of steel, and you’ve got a huge pile of iron ore. You need to refine the ore into iron or steel before you can make something out of it. That metaphor is incredibly accurate for the world of data,” says Matthew Scullion, founder and CEO of Matillion, a cloud data integration and transformation platform.
“We have a gigantic pile of data that’s getting bigger and bigger at logarithmic speed, but you can’t make anything out of it and turn it into analytics-ready data. There’s this huge mountain of possibility that you can’t tap into until you’ve refined it.”
Like those iron workers before the Industrial Revolution, the quality of the output was questionable, the production rate was low and, importantly, only a few people had the skills to do the work. This, says Scullion, is where we find ourselves with data today. This scarcity of skills and processes has created the information gap within organisations.
This information gap is particularly prevalent in large enterprises. The larger the business, the bigger the pile of data that’s valuable but not yet useful. Additionally, there’s more technological complexity across the enterprise, and if you’re a bank or a manufacturer or a huge retailer, only a tiny fraction of the people who work for you will be data analytics experts. This means the problem is more pronounced - and the stakes much higher - in the world’s biggest organisations.
Data, data everywhere…
So what is causing this information gap? Why is there such a disconnect between owning valuable data and turning it into something useful and usable?
There are several reasons. Data may be dispersed across the organisation in silos, out of sight – and mind – of business decision-makers.
There are also technical barriers to companies failing in their efforts to achieve data-driven decision-making. Datasets that live in legacy technologies that can’t keep up with the demand for rapid insights and the diversity, wide distribution, and dynamic nature of modern data. The result: analytics that yield inaccurate and incomplete information, or information that is siloed from the users who need it.
For example, any high street bank will have a computer system where accounts are stored. They will know customer information such as balance, withdrawals and deposits, and any charges. They will also have a customer relationship management system with the customer’s contact details, marketing preferences and anything else they’ve learned through their interactions. Perhaps there is another system that tracks how and when a customer requested help from a service desk, or how many times and in what ways they’ve used online banking or mobile banking. And then finally, there will be information from credit reference agencies about credit histories.
“The problem is that if a bank wants to create a machine learning algorithm to tell them if it’s a good idea to extend credit to a customer, or whether they will appreciate you offering them a certain product, then they need many of those parts of the story in one place,” says Scullion.
The process of making data useful relies on joining it together and organising it so it’s neat and tidy, and aggregated to the right level to make it useful for analytics. But in reality, there can be hundreds or thousands of steps to go from raw material to analytics-ready data. And of course, these steps need to be done carefully. They need to be maintained over time. They need to be secure, to make sure the right data doesn’t get into the wrong hands. And things like permission rules and geographical regulations must be carefully followed.
“On a very small scale, it’s possible to do that without much extra technology to help – you could just write some code and you’ve got the analytics-ready data,” explains Scullion. “But when you scale that up to a large company, then doing it in that manual way isn’t quick, scalable, secure or maintainable enough.
“We can use data to make the world a much better place. But if the pipe supplying us is too small, then the rate at which we can do that is materially reduced. What the world needs and wants is technology that widens that pipe and allows organisations to turn raw data into useful data more quickly.”
Bridging the information gap
What, then, is the answer? “The world needs a technology platform, a highway of data, where all these different bits of raw material can come together and be refined into analytics-ready, trusted, useful, clean data that can then be put to work,” says Scullion.
“We need a technology layer to solve that problem, and that’s what Matillion has built – a data operating system on which enterprises can at scale make that data useful. And in doing so, they can bridge that information gap, fix that constraint in the supply chain of data and make the world a better place by pointing to this new commodity that is data.”
There are now more than 1,000 enterprises around the world using Matillion to bridge their information gap. After launching the platform in late 2015, Matillion has become one of the fastest growing and most valuable late-stage startups in both the UK and Western Europe, driving exciting levels of growth.
Matillion makes use of the limitless, cost-effective power of the cloud. “The cloud has unlocked this generational shift in the use of data,” says Scullion. “Before the cloud, we couldn’t afford, and therefore we didn’t have, the ambition to capture the data and to put it to work. But the cloud provides this incredibly cost effective, powerful and easy-to-make- use-of technology to capture and store data, and then put it to work.
“That’s the backdrop against which the world is pivoting to data and against which data is becoming the new currency.”
Additionally, Scullion says Matillion can be stood up in minutes, and can be learned in a few hours or days. The time to value is a few days or weeks. “That’s because it’s simple to launch, manage and use, but not at the expense of capability,” he says.
“With a technology like ours, which is visual, we call it ‘low code, no code, code optional’. So you can make data useful without needing to write code. That makes it much easier, and more intuitive to use. It also makes it much faster and more productive, but still powerful.”
Matillion is secure, scalable and performant, and can coexist with the enterprise’s rules, policies and procedures, and other technologies. Additionally, the technology can be used by a much broader spectrum of business users, putting the value of the data into the hands of more users.
Q&A: Modern analytics and the business of misinformation
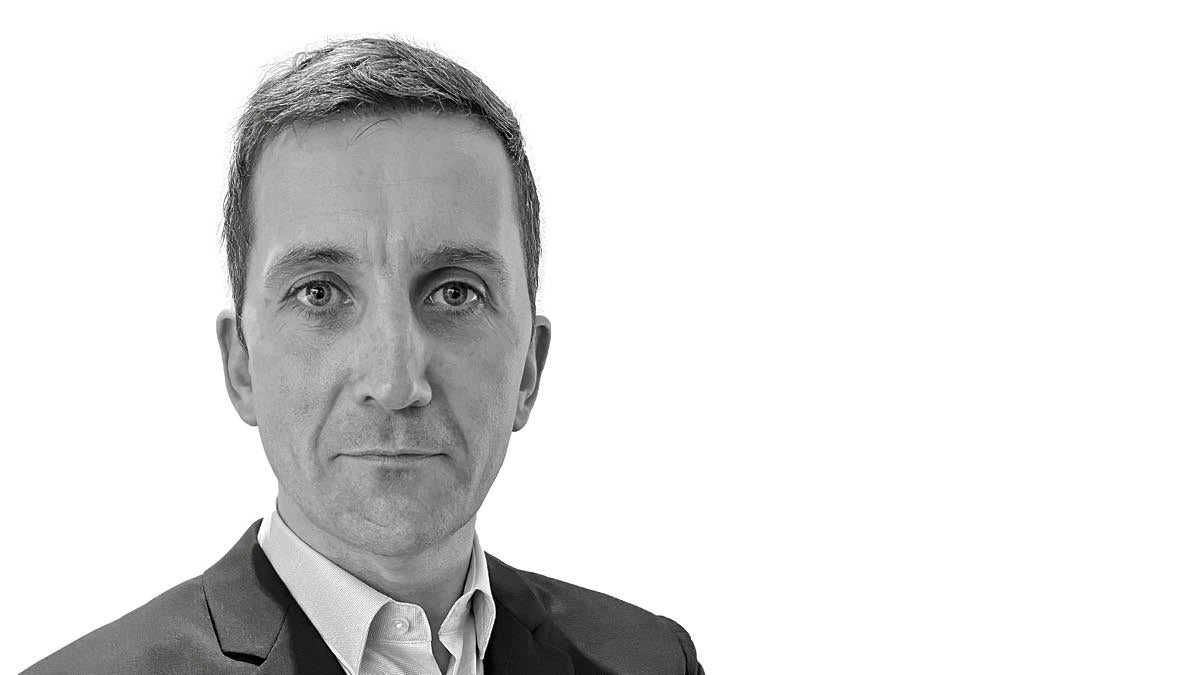
Ciaran Dynes, chief product officer at Matillion on how businesses can make better use of data analytics and the skills they will need to do so
What are some of the challenges facing modern organisations when it comes to data analytics?
A lot of industries evolved with executives that were never required to work with each other; that created artificial barriers. But if everybody is genuinely putting the customer at the heart of their business, there will be no problem with sharing the data. They need to figure out ‘what would a better customer experience be for us?’ And then work out ‘what questions do we want to ask?’ Then it should be the case that the team is required to work together.
That is what happened largely in the big data era. People have realised it’s better to share internal data, and then to pick their battles - the one or two things that they could do to basically improve customer experience, reduce risk, cut costs. It’s possible today because the technology is available to do those things.
Modern analytics is much more about predictiveness; there’s no absolute certainty. Companies must ask questions to build an answer
What is the difference between traditional and modern data analytics?
With traditional analytics you might add up a bunch of numbers to get an answer to a question such as, ‘who are our top 10 customers?’ But with modern analytics, there’s not necessarily one answer. There might be a scale of answers, with a minimum and a maximum, and somewhere between lies the answer.
Modern analytics is much more about predictiveness; there’s no absolute certainty. Companies must ask questions to build an answer. Sometimes the answer will be definitive and sometimes the data comes back and it’s inconclusive. The skill of the business is challenging the answer that is returned, saying ‘I think there’s not enough evidence to suggest that’s the answer.’
What about ‘fake news’ in the context of business data?
You can’t escape the misinformation in society. There’s actually been no rise in misinformation, it’s just digitised nowadays so it gets into the public domain instantly.
Organisations also have misinformation in their business. But misinformation is not always inaccurate information, it is interpretation, it’s context, it’s bias. That’s the real challenge for businesses going forward – they need more and more and more of this different data that we can put together to either prove or disprove that argument to be true or false.
How do we get information? How do we look at misinformation? How do we come up with a question or a hypothesis that may or may not lead to an answer? Modern business is based on those things.
What’s an example of modern analytics?
There are brilliant things that we do in the world with data; there are some amazing stories in government, in healthcare and in insurance. I’m immensely surprised all the time by these interesting stories.
For example, an insurance customer in Washington in the US is looking at the data of forest fires. They provide car, house and property insurance, and with forest fires so prevalent they have an enormous impact on their business. They are pulling data from things like geothermal sensors in the ground and using weather pattern information to perform projections and analysis based on temperature change.
They have no choice but to have an opinion on things like the environment and climate change. That is the business of insurance – hedging bets against a set of outcomes, and some of those outcomes are profound for society.
Learn more about Matillion: matillion.com/demo
Sponsored by
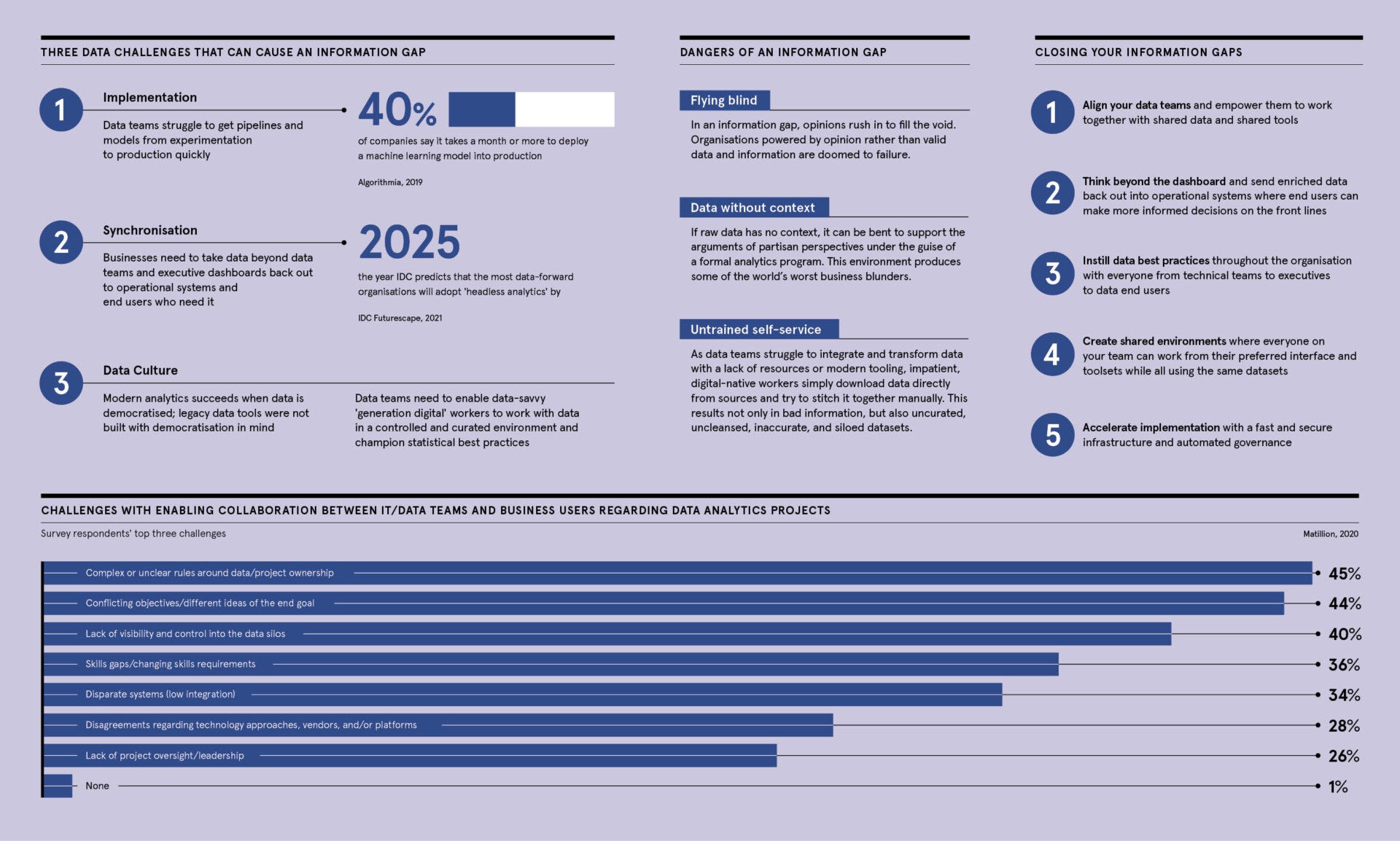
The world’s dependence on data has never been greater. Enterprises rely on analytics to take what are often huge volumes of raw data circulating through the business and transform it into rich, actionable information. However, with many organisations, this isn’t the case.
“Imagine you want to make a tool or a car or a ship or a bridge out of steel, and you’ve got a huge pile of iron ore. You need to refine the ore into iron or steel before you can make something out of it. That metaphor is incredibly accurate for the world of data,” says Matthew Scullion, founder and CEO of Matillion, a cloud data integration and transformation platform.
“We have a gigantic pile of data that’s getting bigger and bigger at logarithmic speed, but you can’t make anything out of it and turn it into analytics-ready data. There’s this huge mountain of possibility that you can’t tap into until you’ve refined it.”