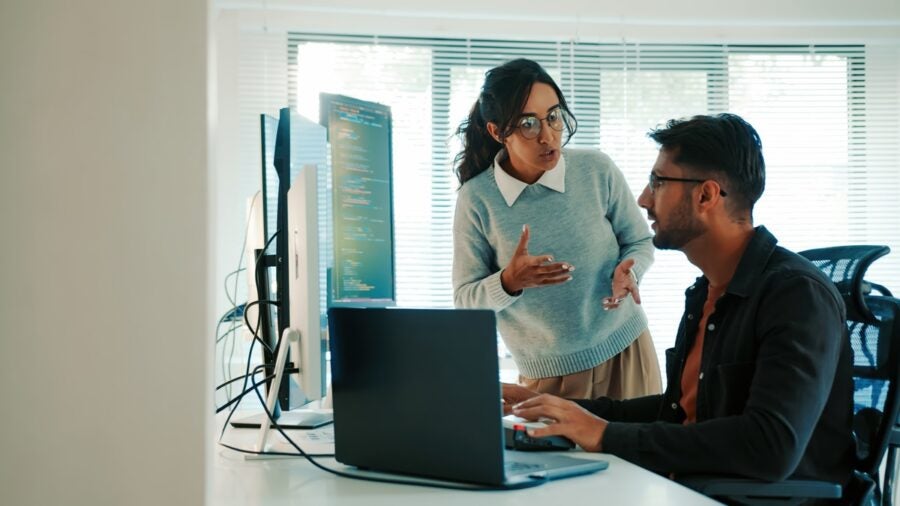
When McDonald’s was forced to remove an AI-powered ordering system from its US drive-through restaurants in June 2024, it served as an example of what can happen when the new technology goes wrong.
The fast food giant’s voice-activated technology began to misinterpret customer orders to comic effect, registering requests for bacon-topped ice cream and hundreds of dollars’ worth of chicken nuggets. Naturally, videos of these gaffes went viral and sparked a torrent of mocking press headlines, forcing the chain to shelve its partnership with IBM, which supplied the ordering system.
The first step to mitigating risks is to take a transparent and traceable approach to model-building
As AI proliferates, such system failures are becoming increasingly common, with organisations including Microsoft, Air Canada, Tesla and Amazon all experiencing their own embarrassing incidents. The costs can be significant in terms of business continuity, brand damage, lawsuits and even regulatory action.
But that hasn’t dampened enthusiasm for AI’s potential. Again, look at McDonald’s: despite the blunder, the company maintains the technology is “part of its restaurants’ future”, highlighting the obvious efficiencies promised by AI solutions.
So how can firms minimise the risks while maximising the benefits?
Three types of AI failures
AI failures can be grouped into three categories. The most common misstep is when an automated system produces an output that is incorrect, biased or even discriminatory. Often the consequence of a system being fed with bad data, this type of failure has led to lawsuits and enforcement actions.
Then there are blunders in data protection. These errors relate to how algorithms are trained and have spawned a spate of recent copyright cases against AI providers such as Microsoft and OpenAI.
The third category of failure is cyber attacks, either against AI systems or facilitated by them, which are becoming more common and more dangerous.
According to Luisa Resmerita, a senior director in the technology segment at FTI Consulting: “The challenge for businesses is striking a balance between the costs of lost opportunities on the one hand and the cost of getting it wrong on the other.”
Developing a robust strategy
Many of the implementation problems may stem from the fact that generative AI is a new technology and is being adopted very quickly. According to the Federation of Small Businesses, 20% of UK small and medium-sized enterprises say they now use some form of AI, but 46% admit they lack the knowledge and/or skills to use it successfully.
A robust AI strategy is key to staying safe. Firms should carry out risk assessments to evaluate the chances and potential consequences of AI system failures. They should also have backups to ensure business continuity; a proper data strategy so that systems are powered with the right information; reliable monitoring processes; and proper human oversight of AI decisions.
An organisation’s approach to governing AI risk must be proportionate to their AI investment strategies and risk tolerance
Executives increasingly require the advice of external partners to ensure their AI strategies are founded on a realistic appraisal of potential risks, according to Stina Connor, an associate director at risk management consultancy Control Risks.
Security teams, on the other hand, often want more tactical support, she explains. Such support could help them understand the trajectory of cyber threats, for example, or to design appropriate policies, guidelines and internal training on the acceptable use of AI within organisations.
Crucially, security and risk mitigation should move in tandem with commercially driven decisions surrounding AI implementation, partnerships and strategies, Connor says.
A responsible culture
Some firms may need to hire dedicated AI and data professionals to oversee AI development and ensure models are ethical, accurate and secure. It is critical that these experts work closely with management, legal and compliance teams so the right AI culture is instilled across the organisation.
Amir Jirbandey, head of growth and marketing at AI-powered video dubbing startup Papercup, notes that larger organisations are increasingly forming dedicated AI committees.
“Groups such as these allow for holistic evaluations of the risks and benefits the technology poses for businesses and their people,” he says.
With coherent regulation in short supply, one of the challenges businesses face is a lack of guidance on AI best practices. This will soon change with the introduction of legislation such as the EU’s AI Act. However, this will in turn see a considerable growth in the compliance burden for internal teams.
Evolving regulations require varying levels of compliance around maintaining detailed documentation and logs of AI systems, notes Mirit Eldor, managing director of life sciences solutions at Elsevier, an information analytics company.
She believes the first step to mitigating risks, including regulatory risk, is to take a transparent and traceable approach to model-building. “This means ensuring AI models are backed by robust data governance, providing visibility into exactly how and what data is being used,” she says.
Innovate, monitor and control
Given the risks, some firms may choose to avoid AI altogether. That would be a mistake, says Michal Szymczak, head of AI strategy at software consultancy Zartis.
It’s best to jump in and experiment. Companies learn through trial and error and are likely to gain a competitive advantage as an early adopter, he says. “No one has the recipe right now, so every company will have to find its own way.”
But it isn’t enough to deploy an AI tool and hope for the best, Szymczak stresses. Firms need a strategy with clear processes for monitoring, notifying and eliminating problems – automatically, if possible.
“A good principle to operate by is ‘innovate, monitor and control’,” he says.
While companies must prepare for possible systems failures, a one-size-fits-all solution is unlikely to work. Some may focus on enhancing corporate governance by injecting AI risk controls into their processes; others will take a more targeted and product-centric approach.
Resmerita thinks AI risk is uniquely multifaceted and therefore must be addressed holistically. “Ultimately, an organisation’s approach to governing AI risk must be proportionate to their AI investment strategies and risk tolerance,” she adds.
Like Jirbandey, she believes executive buy-in is key to building an effective AI strategy that properly accounts for risk. Companies should also define clear roles, responsibilities and protocols for AI governance.
“While defining policy standards is an important first step in the journey, standards are only valuable if they are effectively implemented,” Resmerita concludes.