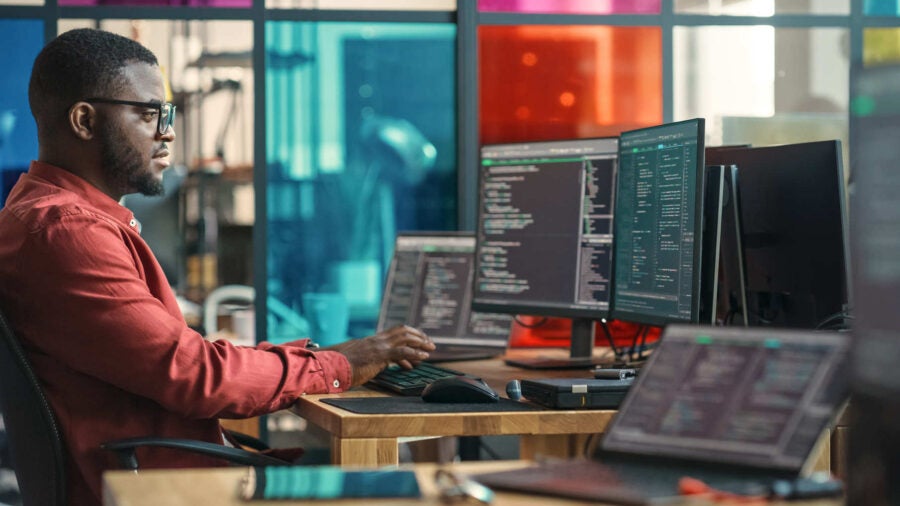
Nearly two decades have passed since British mathematician Clive Humby declared data to be the “new oil”. But, unlike oil, data is not a finite resource. In fact, new data is being created all the time.
And never has it been more important, as thousands of businesses seek to build and train AI algorithms. Deriving success from generative AI means ensuring that the data that shapes it is as good and reliable as possible.
“There’s a kind of black-box thinking around AI at the moment,” says Rachel Aldighieri, managing director of trade body the Data and Marketing Association (DMA). “It’s really important to unpack how AI works: it’s not algorithms that are necessarily causing issues around data privacy and ethics, it’s the data practises companies are using.”
Why responsible data collection matters
Getting these data practises right could provide businesses with a competitive advantage. When discourse is dominated by the behaviour of shady brokers or leaks on the dark web, businesses must show they are acquiring data ethically.
Responsible data collection is becoming an increasingly important differentiator for businesses. Organisations that focus on building digital trust are 1.6 times more likely than the global average to see annual revenue and EBIT growth rates of at least 10%, according to a 2022 survey by McKinsey.
How to make use of internal data
There are many different sources of data which organisations can use to train their AI models. The simplest is a company’s own internal data, garnered from surveys, data-capture forms or customer relationship management (CRM) systems. Firms might have a wealth of information to tap into but they need to be careful about what they use and how.
Any existing data needs to be permissioned for the way organisations wish to use it. When someone initially hands over data, it must be very clear how it will be used. Acquiring new data in a considered way is crucial to protecting brand reputation as well as developing reliable and ethical AI models.
The process begins with obtaining explicit consent. The opt-in process should be easy to understand and transparent. If any personal data is collected, this should be anonymised where necessary.
Understanding what you can and can’t do with data is a vital piece of the ethical AI puzzle
“Data collection processes play a significant role in ensuring ethical practices,” says Stephen Lester, CTO at business services firm Paragon. “Businesses should use transparent methods to collect data, ensuring participants understand how their data will be used and providing appropriate compensation.”
To comply with data protection regulations, businesses should assess risk using legitimate interest tests and data-balancing assessments to ensure they’ve got those permissions.
One challenge is explaining to customers, in a straightforward way, what you’re planning to use their data for, says Aldighieri. Being transparent and accountable upfront, along with having a clearly defined ethical framework, helps to create an auditable trail for data and its permissions, she says. She advises organisations to check the DMA Code to help them create a “principle-led” framework. “If you’re unsure where data has come from, don’t feed it into an algorithm,” she says. “That has to be the bottom line, doesn’t it?”
The challenge of obtaining data ethically
If you don’t have access to data internally, you have three options: use open-source data, buy it from elsewhere or generate it synthetically.
The open data movement has seen everything from census reporting to travel data made available for free. This is a wealth of data that protects personally identifiable information because it is aggregated and anonymised from the start.
However, there are still considerations that must be taken into account for AI modelling. Namely: varying quality and consistency, biases, and the ability to create auditable trails. Although open-source data might be better from a privacy perspective, it can also be less granular and reliable.
For greater granularity, organisations can opt to buy data, but this has its drawbacks too. Data sellers must be thoroughly audited and organisations must ensure they work with reputable brokers.
“You can buy data ethically,” says Chris Stephenson, CTO at data insights consultancy Sagacity. “But you need to define your own ethics and ask the right questions to ensure you’re working with a reputable data seller.”
It’s also important to distinguish between what’s ethical and what’s legal. “Technically what Cambridge Analytica did was legal, but most would agree it wasn’t ethical,” Stephenson says.
Organisations should audit brokers by classifying providers based on their reputation and specialism. Government, academics and well-established commercial data vendors are usually the more reputable sources.
To assess the reputation of providers, look at the organisations that use them as a source. Have university researchers, professional and consultant publications used the provider? Always seek references and cross-check for data quality.
The pros and cons of synthetic data
Then there is synthetic data. This AI-generated data mimics the real thing and may offer a promising alternative. Because it is not attached to real people, privacy protections are baked in and acquiring high volumes of new data is simpler. It can be cheaper too, as businesses won’t need to strike licensing deals with other companies to mine them for data or embark on massive data collection campaigns.
Tens of thousands of data scientists are already using the Synthetic Data Vault by MIT spinout DataCebo to generate synthetic data. The spinout claims that as many as 90% of enterprise use cases could be achieved with synthetic data. And this could well be the year when synthetic data comes into its own. Consulting firm Gartner predicted in 2022 that 60% of all data used to develop AI will be synthetic by the end of 2024.
Sagacity recently used synthetic data to create randomised ‘noise’ – such as misspelt words – within a dataset, in order to train a model to spot mistakes and anomalies.
This was “very useful”, says Sagacity’s Stephenson, but not perfect. “You are often building in bias at the initial stage by how you formulate the data-generation algorithms,” he says.
The firm’s spelling-mistake-spotting model, for example, was based on inputs that the team gave it. “It’s something of a self-fulfilling prophecy,” he says. “That was fine in the context we were using it, because we just wanted to check it could identify specific events, but data quality can be a real issue. The ethics of using it will depend on the context in which the AI is being used.”
Practising continuous data hygiene
Regardless of how and where companies source their data, bias will always be an issue. The data used to train the models will often reflect the bias of its inputters, notes Stephenson. Be that bias which is “introduced programmatically or bias in the sourcing data due to a lack of a representative sample, or just the inbuilt biases of society that we live with every day.”
It is therefore up to whoever is training the model to understand what and where the bias might be – and to take the appropriate steps to tackle it. Aldighieri suggests the effective monitoring of datasets to ensure that only properly permissioned data flows into algorithms.
“Understanding what you can and can’t do with the data is a vital piece of the ethical AI puzzle,” she says. “Organisations need to understand what data they hold, where it is and how it can or can’t be used.”
If you’re unsure where data has come from, don’t feed it into an algorithm
An essential part of reducing bias is ensuring that the teams actually working on artificial intelligence are, themselves, diverse and representative. They must also have an understanding of how to recognise, unpick and challenge bias in automated decision-making.
As part of its approach to building ethics into AI, data analytics firm Qlik created an interdisciplinary council to help advise its internal team and customers. This AI Council “helps guide us, and our customers, through things to think about when setting up AI policies and guidelines,” explains Nick Magnuson, Qlik’s head of AI.
“It was important that we established a council with a cross-disciplinary and cross-functional nature, with people thinking about AI from a whole host of different perspectives,” says Magnuson.
While he says Qlik already had its own foundational policy that put ethics front and centre, the council advises the business on areas that could be strengthened. These services are offered to customers too, to help build trusted foundations for AI that are applied without bias.
Once data collection processes are in place, organisations must continuously monitor their systems to ensure compliance with legal standards as well as their own ethical frameworks. Finally, organisations should establish clear processes for removing content when requested.
AI is not going away and the penalties for unethical or irresponsible AI look set to rise. The best way businesses can protect themselves from this risk is to clean up their data act – and soon.
Ethical data acquisition in a nutshell
Assess what you’re trying to achieve first. Does it actually require artificial intelligence and new data?
This will go some way towards helping to reduce bias at the implementation stage.
Develop your own ethical data framework. Just because something is legal doesn’t mean that it’s ethical.
Data subjects are people – treat them like people. Explain exactly what you’re asking for and allow them to opt in.
Mapping data and being transparent about your use of it, both internally and externally, will help you audit it. Pay attention to the provenance of data.
Models should be scrutinised on an ongoing basis for accuracy, compliance, ethics and bias.
Not everyone is a data scientist. But almost all of us are data subjects. Try to close the knowledge gap by explaining the who, what, when, why and how of your model and its use of data.
Establish a clear and easy path for anyone to opt out of their data being used for AI.
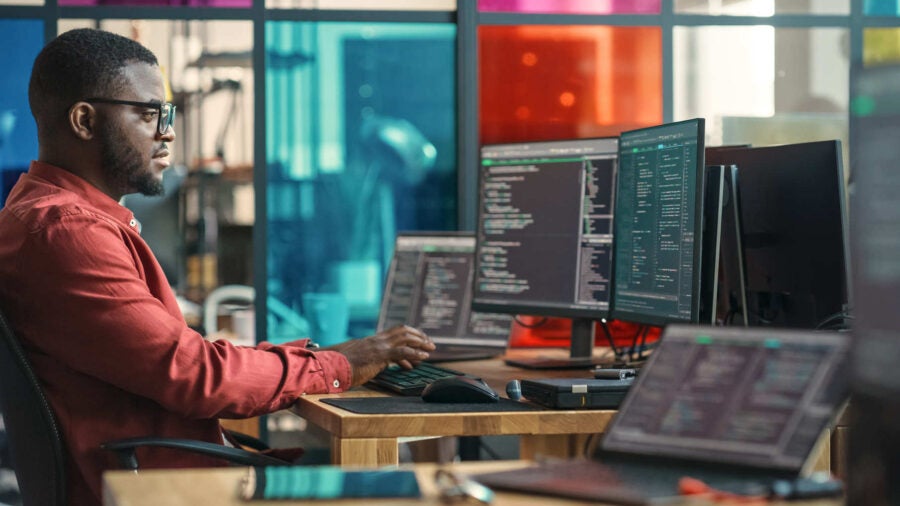
Nearly two decades have passed since British mathematician Clive Humby declared data to be the “new oil”. But, unlike oil, data is not a finite resource. In fact, new data is being created all the time.
And never has it been more important, as thousands of businesses seek to build and train AI algorithms. Deriving success from generative AI means ensuring that the data that shapes it is as good and reliable as possible.
“There’s a kind of black-box thinking around AI at the moment,” says Rachel Aldighieri, managing director of trade body the Data and Marketing Association (DMA). “It’s really important to unpack how AI works: it’s not algorithms that are necessarily causing issues around data privacy and ethics, it’s the data practises companies are using.”