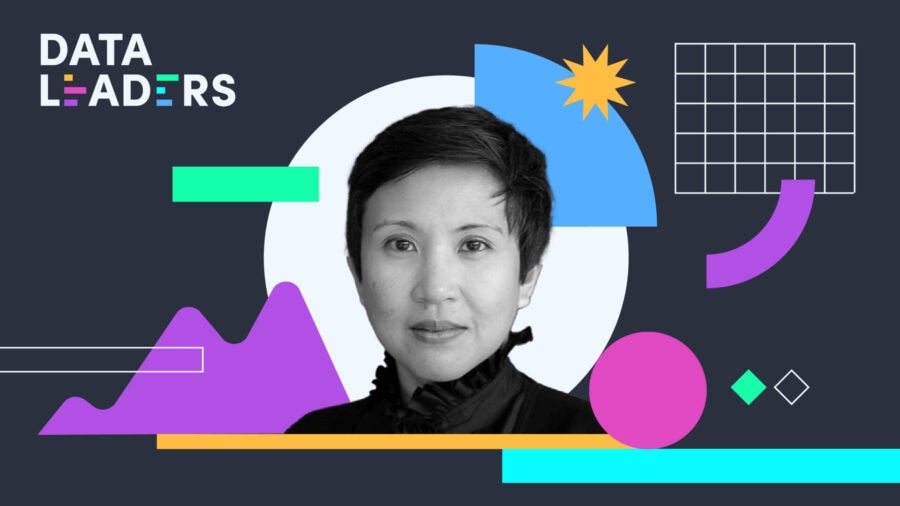
The race to embrace AI is well and truly on. Organisations spanning countless industries are under pressure to keep up with competitors and satisfy board members by making innovative use of GenAI. At the same time, more established forms of AI and machine learning are still underutilised by many firms. So what separates the front-runners from the laggards?
Often it’s how their data is structured, accessed and governed. “As Informatica CEO Amit Walia said recently, everything is ready for AI except your data,” says Ling Ling Lo, global head of data strategy and transformation and EMEA head of Nomura’s chief data office. “Everyone wants to do innovation, but there are certain foundational things that you need to have in place to innovate and scale.”
Data governance should evolve in parallel with an AI initiative
Indeed, three common problems occur when attempting to deploy and scale AI. “The first problem that firms normally have is that they’re not sure what data is needed,” says Lo. “And the second is that they know what data is needed, but they can’t get to it because it’s buried somewhere. The third is that the data is available, but it’s hard to use – either because there’s a format problem, a quality problem or there’s not enough of it.”
A Nomura employee recently encountered the ‘not enough data’ issue while working on an anomaly detection project. “They had one commonly known data set for it,” Lo explains. “But what they hadn’t appreciated is that two other data sets were needed to do this anomaly detection exercise because it’s partly about correlation. And these were quite hard to get.”
The fact that some of the necessary data was lacking only became clear once the project was underway. This meant it had to be put on hold until the additional data could be obtained and stored for a few months. “This is quite common,” says Lo, “and it’s the easiest way to kill off an AI use case.”
Data governance and management
A more fluid and flexible approach to data governance may also be needed in the AI era. “This technology is emerging faster than governance and controls processes can keep up with, and so some theoretical approaches – e.g. sort out your data governance before you explore AI – don’t really work in real life,” says Lo.
Data governance should therefore evolve in parallel with an AI initiative. This more emergent approach begins with an AI use case, perhaps some experimentation – then if the project stalls because the right governance processes aren’t in place, teams go back and address this before continuing. “Sometimes innovation has to live in a fairly unstructured space before you then put in the guardrails,” Lo argues.
When it comes to data management, the approaches and processes needed for the successful adoption and scaling of AI are no different though. “If you had data quality problems before, guess what? You’ll still have a data quality problem with AI – and it could be significantly augmented,” says Lo.
Some financial services firms are struggling to address these issues and access the data they need to make the most of AI and machine learning. “It’s a combination of legacy architecture and a culture where certain types of data are deemed more important than others,” Lo explains. “Therefore the data management focus is on those types of data as opposed to the ones you really need now for machine learning, which tends to be raw, atomic-level data.”
The need for ‘purple people’
To underline the kind of organisational culture that will drive successful AI projects, Lo points to the thought leader Wayne Eckerson’s ‘purple people’ analogy. He described two personality types – red and blue – that are traditionally divided within most organisations. Purple people are those who hold characteristics from both, which helps them and the organisation excel.
“In any organisation, you have people that are tech savvy – the blue people – and you have people who are very focused on revenue generation, the business domain side of things – the red people,” says Lo.
While blue people naturally tend to gravitate toward data and AI literacy programs, an awareness of data quality, tagging and other related issues is becoming even more important for red types because GenAI is such a democratic tool. “You don’t need to have technical skill to use it, so the risk of actually getting something wrong is far greater,” says Lo.
However, she feels it is actually the red people who are currently becoming more ‘purple’ overall. “They [blues] are very stuck in their domain. And so while we talk a lot about data literacy, I think the reverse is also true: people who are already data literate need to gravitate more toward having domain knowledge outside of the data and tech sphere.”
An organisation that has more of these ‘purple people’ should find that its data and AI strategy is better aligned with business goals, ultimately leading to better results from any investment in AI. “You want that hybrid perspective to make sure your solutions are business appropriate – that they solve what’s being asked for and more,” says Lo.
Interestingly, Lo herself would have defined herself as a red person before changing tack roughly four years ago. “My core skillset was actually in financial planning and strategy, so when I moved into data it was a bit of a shock to the system initially. But it was also interesting because one of the things that I observed was the disconnect between data and business.”
As such, her biggest takeaway when she joined the data profession was that while technical skills are important, they need to be coupled with very strong commerciality. “That has informed how I structure my team and the sort of individuals I bring into it – particularly the ones that are business-facing,” she says.
As firms start to think more deeply about how they want to use AI and the areas of the business where it will have the most impact, it will “inevitably shine a light on whether they have enough of the data that’s needed,” says Lo. “Because to do AI well, you need to do data well.”
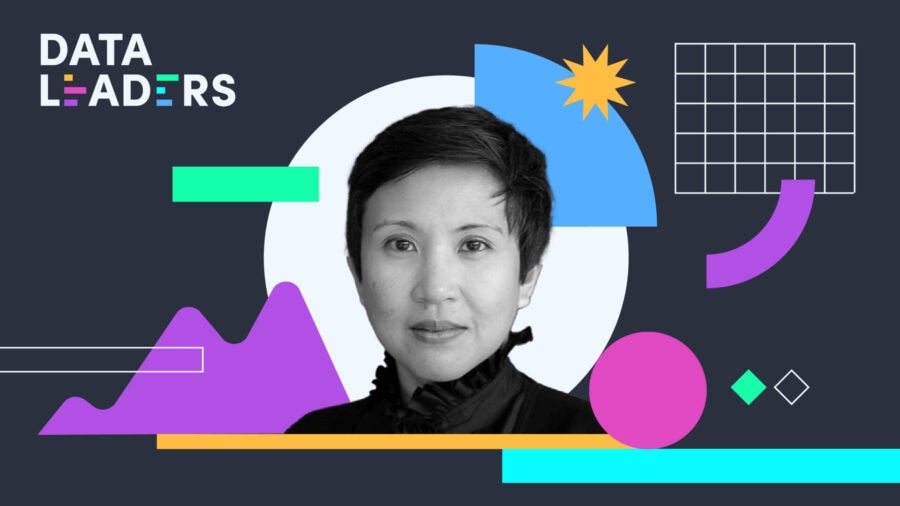
The race to embrace AI is well and truly on. Organisations spanning countless industries are under pressure to keep up with competitors and satisfy board members by making innovative use of GenAI. At the same time, more established forms of AI and machine learning are still underutilised by many firms. So what separates the front-runners from the laggards?
Often it’s how their data is structured, accessed and governed. “As Informatica CEO Amit Walia said recently, everything is ready for AI except your data,” says Ling Ling Lo, global head of data strategy and transformation and EMEA head of Nomura's chief data office. “Everyone wants to do innovation, but there are certain foundational things that you need to have in place to innovate and scale.”
Data governance should evolve in parallel with an AI initiative