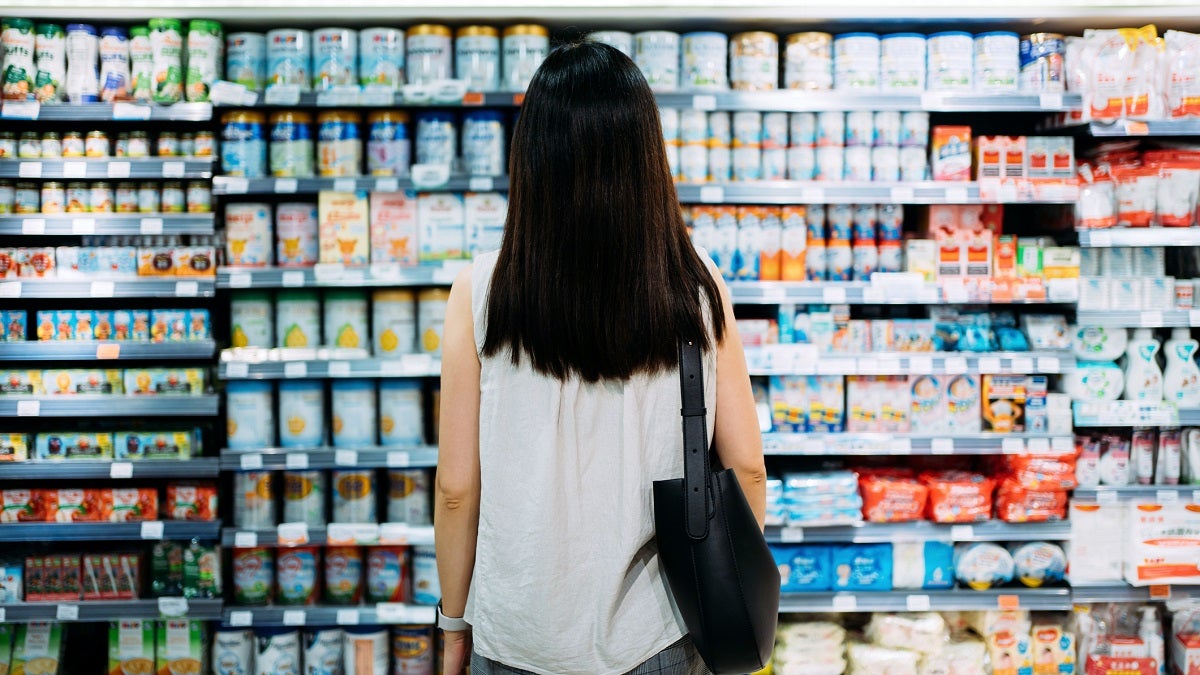
“A lot of times, people don’t know what they want until you show it to them.”
These words, spoken in 1997 by the late Apple co-founder Steve Jobs, have been reverberating through the corridors of consumer-facing businesses ever since. Indeed, virtually all online shoppers can expect to encounter a digital enterprise that suggests what goods and services they’re likely to want.
Such pointers are powered by recommendation engines, pioneered by Amazon and Netflix, which are increasingly using AI technology such as machine learning to predict what any given customer is most likely to consume, based on their purchase history and any other relevant data that’s available.
As the technology underpinning these engines has improved greatly in recent years, so has the quality of their recommendations. This has helped etailers and online brands using the direct-to-consumer channel to increase the ‘stickiness’ of their websites and encourage repeat business.
Paul Hornby is director of the digital customer experience at the Very Group, which operates clothing and homeware retailer very.co.uk. He believes that there is a clear business case for using such systems.
We create space for human recommendations as well as algorithmic ones. Having both is key for us
“Recommending content that is personalised creates a more contextually relevant journey for each customer and a better experience overall,” Hornby says. “For us, well-executed recommendations help customers to find the right products.”
This, he adds, increases both the conversion rate (the percentage of website visits that end in a transaction) and the average value of each order.
D2C brands that use recommendation engines stand to gain a competitive edge over those that don’t, according to Ronan Tighe, chief product officer at Moonpig. For the provider of personalised greeting cards, “this is all about removing friction for customers so that they’ll come to us rather than any other provider”, he says.
The goal, therefore, is for “any brand to be able to say that it knows each customer better than anybody else”, argues Michael Schrage, a research fellow at Massachusetts Institute of Technology and the author of Recommendation Engines. Those that do will succeed – and those that don’t risk falling by the wayside, he warns.
Limiting customer choice
But the growing adoption of recommendation engines has inevitably prompted concerns that the technology limits choice for customers. Some critics of their use say that the joy of the unexpected chance purchase online will soon be a thing of the past.
“The whole notion of how you build in novelty and serendipity is a core research priority,” Schrage says. “Imagine how horrible it would be if there were an ice cream recommender that suggested only different types of vanilla: vanilla with sprinkles, vanilla with choc chips and so on. This is one of the most important issues that must be tackled.”
Hornby agrees that it’s important to acknowledge and address such issues. “Our challenge is to ensure that we don’t place customers inside an echo chamber. We need to keep offering them fresh choices in a helpful way,” he says.
Sanjeevan Bala is group chief data and AI officer at ITV, whose digital streaming service, the ITV Hub, uses recommendation engines. He notes that there will be a human element in the suggestions made by this service for the foreseeable future.
“We create space for human recommendations as well as algorithmic ones. Having both is key for us,” Bala says. “When you visit the ITV Hub website, the first thing you’ll see is a space that we call the rail. We ensure that someone with an editorial mindset decides what appears here. That gives us those ‘serendipity’ moments. You could be a Coronation Street fan, for instance, and you’re only really interested in watching that programme. But then something else might drop in front of you. You might think of giving that a go, or you might not – it’s fine either way.”
Human input is less important to Moonpig. It takes a different approach to recommendations, Tighe says, because its customers are “always buying for someone else. We’re using all the data and insight that we have from individuals who are similar to them. Some customers are buying for people they’re very close to, so they’ll know exactly what they want anyway. But, if a grandmother is buying for her grandson, say, she won’t necessarily know what a 10-year-old boy likes. We therefore view the data as increasing the range of options that people can think of. Our use of recommendations actually expands your choice instead of narrowing it.”
Hornby has drawn similar conclusions at Very, having discovered that its systems will nearly always outperform humans.
“Making AI-powered recommendations feel ‘human’ is one of the exciting challenges we’re facing,” he says. “At one point we did have humans manually curating recommendations to ensure that we were displaying credible outfits that aligned well with our visual merchandising. The results looked great, but when we then tested these against the ones produced by algorithms, the algorithms won. We’re now back to understanding how we can use AI to create highly relevant recommendations at a category level.”
Creating a relevant customer experience
So are we about to enter an etail world lacking in the joys of the random purchase? Emily Pfeiffer, a senior analyst at research and advisory firm Forrester, offers two reasons why it’s important not to overstate the problem.
First, despite the Covid-induced ecommerce boom, bricks-and-mortar retail – and the serendipitous shopping experiences that this can deliver – will continue to dominate. “We predict that physical stores will still be accounting for three-quarters of retail sales in western Europe by 2025,” Pfeiffer says.
Second, it boils down to the kind of goods or services being offered online and each consumer’s intent. “It depends on the individual,” she says. “There are some people who don’t want to have a shopping ‘adventure’ and stumble across new things. They know exactly what they’re seeking and they will be annoyed if they’re recommended something they don’t want, feeling that the brand doesn’t understand them well enough. But there are also people who want to be shown the things that they may not have considered.”
Pfeiffer continues: “On the one hand, you might be taking away the adventure and crowding out the things that might have been discovered. On the other, if you don’t create a relevant experience, you lose the customer. The risk of limiting choice is not as great as the risk of losing your customer because you’re not creating that relevance.”
Ultimately, Schrage argues, the effective use of recommendation engines requires businesses to change their mindset and consider how the technology can deliver agency for consumers.
“The key thing is that these are recommendation systems, not compliance systems,” he says. “For all those executives and MBA-holders raised on the notion that they need to give customers the right answer, this presents a cultural and psychological conflict. Recommenders aren’t about giving the right answer; they’re about offering the right choices.”
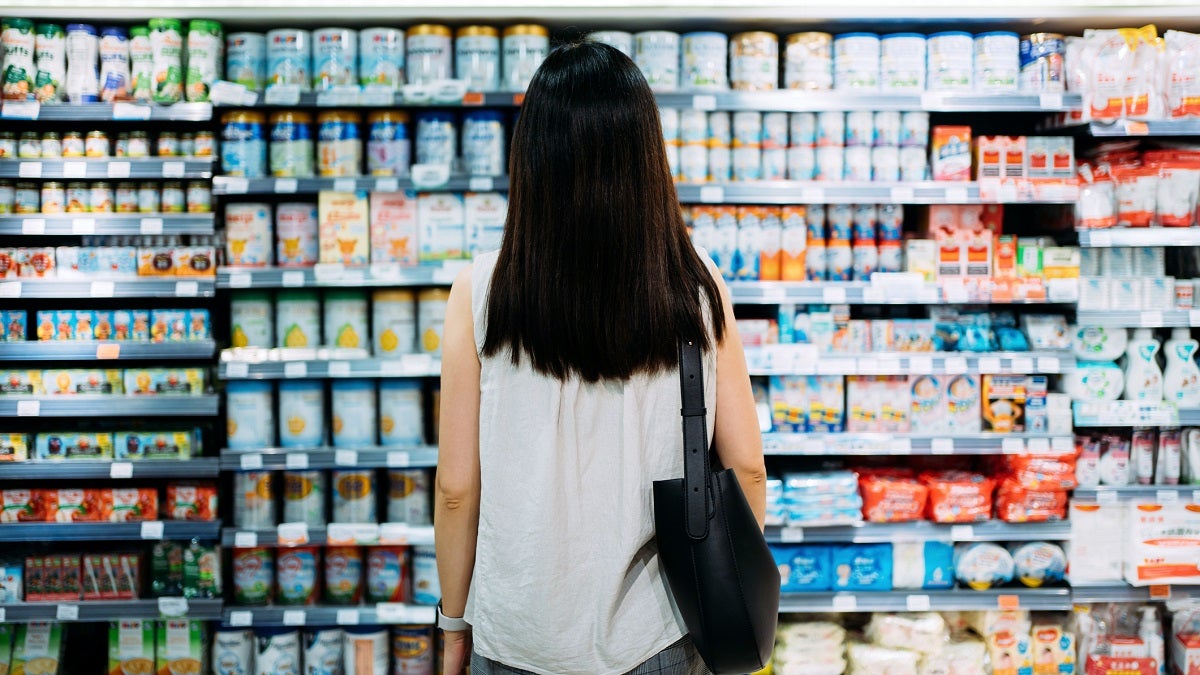
“A lot of times, people don’t know what they want until you show it to them.”
These words, spoken in 1997 by the late Apple co-founder Steve Jobs, have been reverberating through the corridors of consumer-facing businesses ever since. Indeed, virtually all online shoppers can expect to encounter a digital enterprise that suggests what goods and services they’re likely to want.
Such pointers are powered by recommendation engines, pioneered by Amazon and Netflix, which are increasingly using AI technology such as machine learning to predict what any given customer is most likely to consume, based on their purchase history and any other relevant data that’s available.