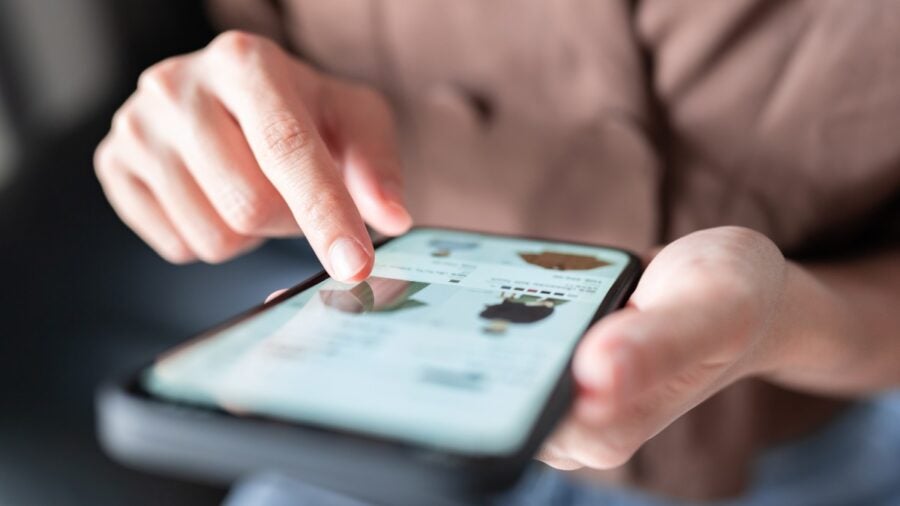
When shoppers land on Puma’s homepage this Christmas, what they’ll see is the result of almost a year’s work by the sports retailer.
Like many other brands, Puma needs to order its products two seasons in advance, but the company also spends months perfecting its range management strategy for peak trading periods like Christmas. It’s all part of an effort to show customers the right product at the right time, to increase the chances of converting visitors into buyers.
It’s also a question of avoiding a common pitfall to which many retailers succumb: simply showcasing their best-selling products or focusing on big discounts and promotions – a strategy which is likely to appeal to certain customers more than others. It can also leave retailers with a big pile of expensive inventory after Christmas, as products which are less recommended are left unsold.
Modern range management is a delicate balancing act. “Successful range management is about how you maximise profit from the products you have in inventory, and what levers you can pull to get those products sold without overspending on digital marketing or having to discount inventory and basically give stuff away,” says Michael Ross, senior vice-president at retail consultancy Edited.
To get it right, retailers must understand exactly what the customer wants. And they also need to know what the customer might buy, even though they didn’t necessarily know they wanted it, adds Ross. “Probably 80% of range management is helping the customer find the things they want, but the other 20% is about recommendation and cross-selling, and that can be where retailers make the most profit,” he says.
At Groupon, this idea is expressed as a balance between ‘exploration’ and ‘exploitation’. “We can exploit what we already know about the customer, but that has to be balanced with exploring and showing the customer other categories and new products that we’ve got,” says Dan Arden, Groupon’s global CRM and marketing operations director. “If 80% of customer revenue is from one category, we want to use that other 20% to show them things that might be higher-margin or a strong cross-selling opportunity,” he says.
The problem with personalisation
One increasingly common way to go about this is personalisation. Indeed, Puma is typical of a growing number of retailers using AI models to capture and analyse vast quantities of customer data to allow for tailored recommendations on its homepage. But personalisation is not the be-all and end-all. “It’s a combination of personalised recommendations to the customer based on the AI insights, plus some brand messaging and campaigns we want to promote,” says Dylan Valade, head of technology in the global ecommerce team at Puma.
We can exploit what we already know about the customer but that must be balanced with exploring and showing the customer other categories and new products
When someone visits the Puma website, the site personalises content based on AI-driven assumptions about the customer’s past actions and likely interests. The system also tracks inventory levels, promoting products that are in stock across all sizes over and above those that are already selling out.
But given stringent data protection laws in the EU, Puma recognises that adapting the online range in this way is becoming more difficult. Instead, the company is increasingly focusing on segmenting customers into certain audience groups, based on common behaviours. “Getting enough data to have a personalised message for each shopper is a lot of effort, so we’re better off looking for audience groups with common interests and goals,” says Valade. “From a data privacy point of view, it’s better.”
Groupon is taking a similar approach. This Christmas, visitors to its website will see a combination of the top 10 best-selling items and personalised recommendations based on their previous behaviour and buying habits. This works best, however, if the customer has provided lots of data and returns to the site regularly. “The problem with algorithms is they need feeding almost constantly and if there isn’t constant data, they can struggle,” says Arden.
This year, Groupon has invested heavily in the creation of segmented customer persona models, which its algorithm can use to identify the likely preferences of customers based on smaller amounts of data. “If there are limited signals from a customer, then we can look at what data we have – perhaps the last deal the customer looked at, and what email link they clicked – and then look at the models to see what we know about other customers who exhibit those behaviours. We can often say with a high degree of confidence that most customers that do X went on to do Y, even without knowing that individual,” explains Arden. He adds that this partly comes from the use of social listening tools and Google Trends, which help Groupon identify what people are posting about and when.
The human touch
But these kinds of technologies will only work if the underlying strategy is sound, cautions Ross. “Where many businesses go wrong is saying, ‘Oh, we need to do some AI’, but that’s a solution in search of a problem. What’s more interesting is to ask what the business problem is, and how we can use data to make better, more intelligent decisions.”
Ross cites the example of a retailer which was using AI to provide recommendations to website visitors. The recommendations were delivering a high conversion rate and appeared to be successful. “However, when we looked at the data, only 11% of products were ever recommended and the business had $100m (£83m) in slow-moving inventory,” he says. “The real question is how we can use recommendations to help customers but with a preference for those areas where we have more inventory.”
Groupon’s Arden views technological options in the same way as the autopilot function on a plane. “You use autopilot but if you’re flying into a storm or there’s a risky situation, the pilot takes over,” he says. “Data is great, but retailers are looking at three years of data where nothing was normal, so this Christmas it’s essential that we apply human common sense at the same time.”